As AI and machine-learning tools become more pervasive and accessible, product and engineering teams across all types of organizations are developing innovative, AI-powered products and features. AI is particularly well-suited for pattern recognition, prediction and forecasting, and the personalization of user experience, all of which are common in organizations that deal with data.
A precursor to applying AI is data — lots and lots of it! Large data sets are generally required to train an AI model, and any organization that has large data sets will no doubt face challenges that AI can help solve. Alternatively, data collection may be “phase one” of AI product development if data sets don’t yet exist.
Whatever data sets you’re planning to use, it’s highly likely that people were involved in either the capture of that data or will be engaging with your AI feature in some way. Principles for UX design and data visualization should be an early consideration at data capture, and/or in the presentation of data to users.
1. Consider the user experience early
Understanding how users will engage with your AI product at the start of model development can help to put useful guardrails on your AI project and ensure the team is focused on a shared end goal.
If we take the ‘”Recommended for You” section of a movie streaming service, for example, outlining what the user will see in this feature before kicking off data analysis will allow the team to focus only on model outputs that will add value. So if your user research determined the movie title, image, actors and length will be valuable information for the user to see in the recommendation, the engineering team would have important context when deciding which data sets should train the model. Actor and movie length data seem key to ensuring recommendations are accurate.
The user experience can be broken down into three parts:
- Before — What is the user trying to achieve? How does the user arrive at this experience? Where do they go? What should they expect?
- During — What should they see to orient themselves? Is it clear what to do next? How are they guided through errors?
- After — Did the user achieve their goal? Is there a clear “end” to the experience? What are the follow-up steps (if any)?
Knowing what a user should see before, during and after interacting with your model will ensure the engineering team is training the AI model on accurate data from the start, as well as providing an output that is most useful to users.
2. Be transparent about how you’re using data
Will your users know what is happening to the data you’re collecting from them, and why you need it? Would your users need to read pages of your T&Cs to get a hint? Think about adding the rationale into the product itself. A simple “this data will allow us to recommend better content” could remove friction points from the user experience, and add a layer of transparency to the experience.
When users reach out for support from a counselor at The Trevor Project, we make it clear that the information we ask for before connecting them with a counselor will be used to give them better support.
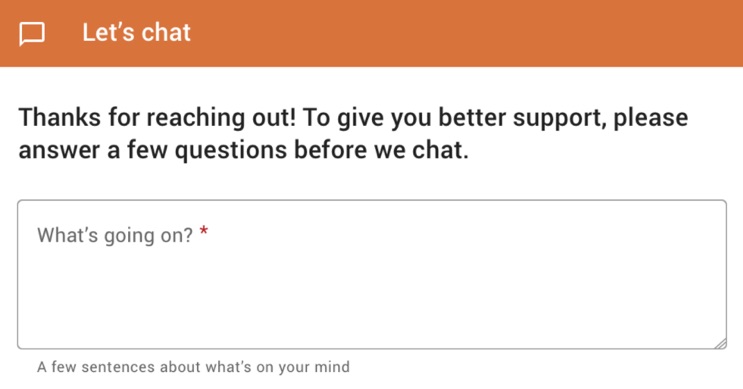
Image Credits: Trevor Project (opens in a new window)
If your model presents outputs to users, go a step further and explain how your model came to its conclusion. Google’s “Why this ad?” option gives you insight into what drives the search results you see. It also lets you disable ad personalization completely, allowing the user to control how their personal information is used. Explaining how your model works or its level of accuracy can increase trust in your user base, and empower users to decide on their own terms whether to engage with the result. Low accuracy levels could also be used as a prompt to collect additional insights from users to improve your model.
3. Collect user insights on how your model performs
Prompting users to give feedback on their experience allows the Product team to make ongoing improvements to the user experience over time. When thinking about feedback collection, consider how the AI engineering team could benefit from ongoing user feedback, too. Sometimes humans can spot obvious errors that AI wouldn’t, and your user base is made up exclusively of humans!
One example of user feedback collection in action is when Google identifies an email as dangerous, but allows the user to use their own logic to flag the email as “Safe.” This ongoing, manual user correction allows the model to continuously learn what dangerous messaging looks like over time.
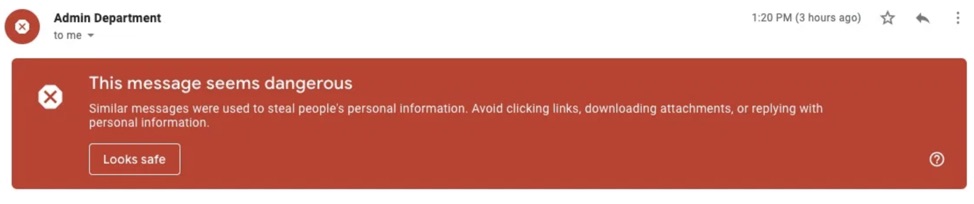
Image Credits: Google
If your user base also has the contextual knowledge to explain why the AI is incorrect, this context could be crucial to improving the model. If a user notices an anomaly in the results returned by the AI, think of how you could include a way for the user to easily report the anomaly. What question(s) could you ask a user to garner key insights for the engineering team, and to provide useful signals to improve the model? Engineering teams and UX designers can work together during model development to plan for feedback collection early on and set the model up for ongoing iterative improvement.
4. Evaluate accessibility when collecting user data
Accessibility issues result in skewed data collection, and AI that is trained on exclusionary data sets can create AI bias. For instance, facial recognition algorithms that were trained on a data set consisting mostly of white male faces will perform poorly for anyone who is not white or male. For organizations like The Trevor Project that directly support LGBTQ youth, including considerations for sexual orientation and gender identity are extremely important. Looking for inclusive data sets externally is just as important as ensuring the data you bring to the table, or intend to collect, is inclusive.
When collecting user data, consider the platform your users will leverage to interact with your AI, and how you could make it more accessible. If your platform requires payment, does not meet accessibility guidelines or has a particularly cumbersome user experience, you will receive fewer signals from those who cannot afford the subscription, have accessibility needs or are less tech-savvy.
Every product leader and AI engineer has the ability to ensure marginalized and underrepresented groups in society can access the products they’re building. Understanding who you are unconsciously excluding from your data set is the first step in building more inclusive AI products.
5. Consider how you will measure fairness at the start of model development
Fairness goes hand-in-hand with ensuring your training data is inclusive. Measuring fairness in a model requires you to understand how your model may be less fair in certain use cases. For models using people data, looking at how the model performs across different demographics can be a good start. However, if your data set does not include demographic information, this type of fairness analysis could be impossible.
When designing your model, think about how the output could be skewed by your data, or how it could underserve certain people. Ensure the data sets you use to train, and the data you’re collecting from users, are rich enough to measure fairness. Consider how you will monitor fairness as part of regular model maintenance. Set a fairness threshold, and create a plan for how you would adjust or retrain the model if it becomes less fair over time.
As a new or seasoned technology worker developing AI-powered tools, it’s never too early or too late to consider how your tools are perceived by and impact your users. AI technology has the potential to reach millions of users at scale and can be applied in high-stakes use cases. Considering the user experience holistically — including how the AI output will impact people — is not only best-practice but can be an ethical necessity.
https://ift.tt/2CXEJUF Five ways to bring a UX lens to your AI project https://ift.tt/2CxyqY8
0 comments
Post a Comment